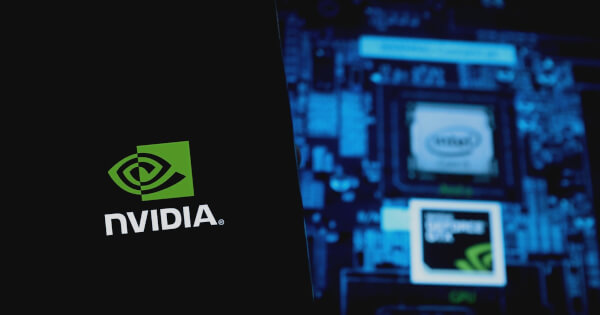
Zach Anderson
May 21, 2025 19:00
NVIDIA’s Isaac Lab is revolutionizing robotic assembly with its sim-to-real transfer capabilities, enhancing adaptability and precision in industrial applications. Discover how this technology is shaping the future of robotics.
In the ever-evolving field of robotics, NVIDIA Isaac Lab is making significant strides by bridging the gap between simulation and real-world applications. According to NVIDIA, this advancement is particularly impactful in industrial sectors such as manufacturing, automotive, aerospace, and medical devices, where precision and adaptability are paramount.
Challenges in Robotic Assembly
Robotic assembly, despite its critical role across various industries, remains a challenging endeavor. The complexity arises from the need for robots to manipulate objects through continuous physical contact, demanding high precision and accuracy. Traditional robotic systems have been limited by fixed automation, which requires extensive human engineering for specific tasks, thereby restricting scalability and adaptability.
Advancements with NVIDIA Isaac Lab
NVIDIA is addressing these challenges through flexible automation, integrating robotics with simulation and artificial intelligence. The company has been advancing research in this area for several years, collaborating with partners like Universal Robots to translate research innovations into practical industrial applications.
One of the key innovations is the zero-shot sim-to-real transfer of a gear assembly task on the UR10e robot, a task designed and trained in NVIDIA Isaac Lab and deployed using NVIDIA Isaac ROS. Isaac Lab, an open-source training framework, and Isaac ROS, a collection of accelerated computing packages, provide the necessary tools for developing transferable robotic skills across diverse environments.
Simulation to Reality: The Workflow
The process involves training robots in a simulated environment using reinforcement learning (RL), a technique that allows learning through trial and error across multiple parallel environments. This approach makes it feasible to simulate complex interactions that were previously computationally intractable.
Isaac Lab supports both imitation learning and RL, offering flexibility in training approaches. The simulation environment enables the training of core skills such as grasp generation, motion generation, and insertion, which are essential for tasks like gear assembly.
Real-World Implementation
In collaboration with Universal Robots, NVIDIA has successfully demonstrated the deployment of RL-trained policies on real-world robots using a torque control interface. This interface allows for safe and compliant interactions, enhancing the adaptability of robotic systems in real-world settings.
The deployment involves a perception pipeline that estimates gear poses, which are then used to predict joint positions for the robot, enabling precise task execution. The trained policies have shown robustness in assembling gears placed in random positions, demonstrating the effectiveness of the sim-to-real transfer.
Future Prospects
NVIDIA’s continued efforts in enhancing robotic assembly through advanced simulation techniques and AI are paving the way for more adaptable and scalable robotic systems. The company’s work in this domain not only showcases the potential of robotics in industrial applications but also sets the stage for further innovations in the field.
For more detailed insights, visit the NVIDIA blog.
Image source: Shutterstock